Training and Education for AI-enabled Weapons Systems - Joseph O. Chapa
- Nomos Foundation
- Mar 25
- 7 min read
Special Insight Article by Joseph O. Chapa, Author of 'Is Remote Warfare Moral' (2022) and Faculty, US Air Force, Marine Command and Staff College.*
What counts as “drone warfare” is a moving target. Not long ago, at the height of the US’s and its allies’ operations in Afghanistan and Iraq, the term “drone warfare” referred to multi-million dollar Predator and Reaper aircraft releasing laser- or global position system- (GPS) guided munitions. Aircraft might have flown 14, or 18, or 22 hour-long sorties before releasing their ordinance and returning to large-scale air bases to refuel.
Today, drone warfare in Ukraine is very different. First person view (FPV) drone operators who, before the war, were police officers, sales managers, and film makers.
As both technology and tactics develop at a pace only war can explain, it can be difficult to know what ethical questions these novel ways of war might raise.
Here, I want to focus on one moral question in particular that arises from modern approaches to AI-enabled drone warfare. It is a human problem, rather than a technological one. In the literature on technology ethics, it is often called automation bias—"the tendency to over-rely on automation.” But the phenomenon is much broader than automation and much deeper than technology. Ultimately, automation bias is just one instance of overconfidence in one’s own epistemic position. The answer must be right, we think, because we lack access to the information that would show us that the answer is wrong.
Before I turn to the history of AI, let me show you an example of this problem of epistemic overconfidence in a context that has much to do with technology and war, but nothing to do with AI.
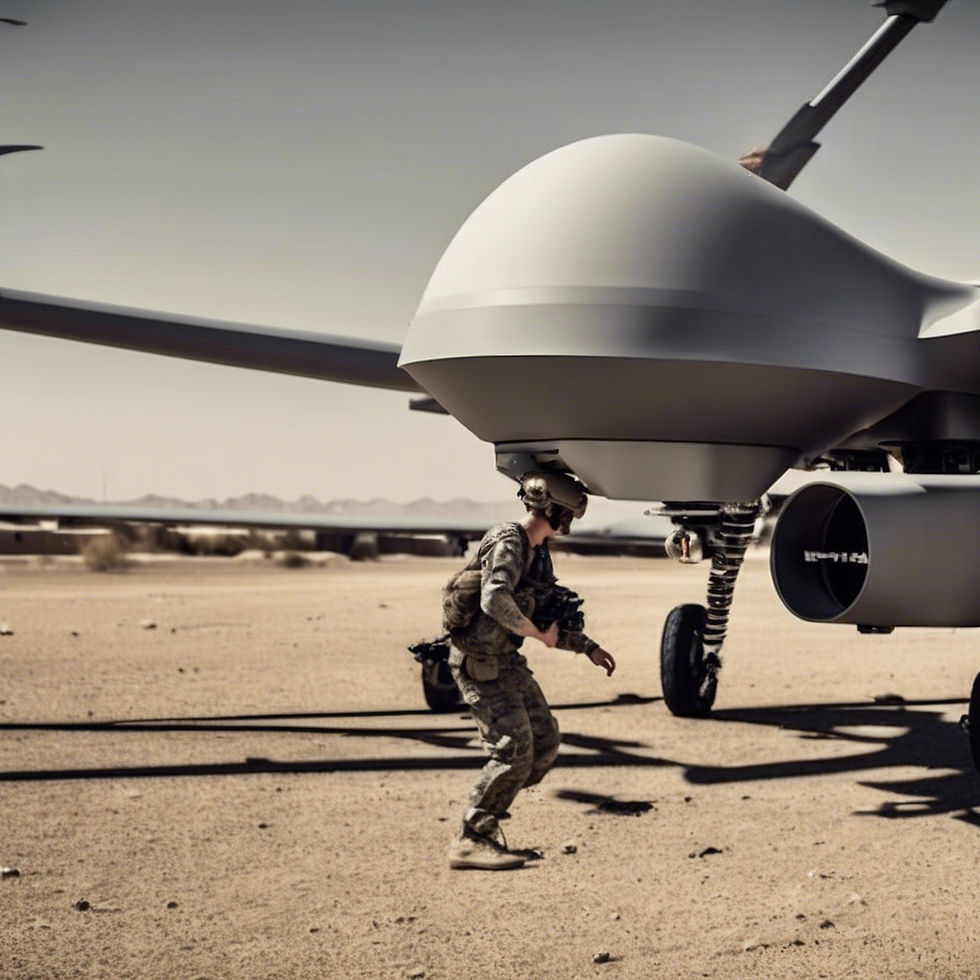
In 2011, the Los Angeles Times submitted a Freedom of Information Act request to obtain the chat logs of a US Air Force MQ-1 Predator mission over Afghanistan. That transcript has since been printed in the newspaper, but it has also been reprinted in several books and other accounts.
The devastating transcript provides the Predator crew’s running commentary that explains how they convinced themselves that the people under their crosshairs were terrorists, though in fact, the people under their crosshairs were families on their way to market. In aircraft safety, the “chain of events” is a well-known analogy for the series of steps aircrew and air traffic controllers take that can ultimately result in a fatality incident. The analogy is apt because one need not destroy the whole chain to prevent the mishap, one need only break one link in the chain. Similarly in the Predator chat logs, to prevent the travesty, the crew would not have had to correct every one of the misapprehensions nor seek clarification on every one of their assumptions. They would have had to break just one link in the chain.
Ultimately, the crew convinced themselves of the terrorists under their crosshairs and called in a two-ship of US Army Kiowa helicopters to attack the innocent families on their way to market. When the devastating truth of what had happened descended upon the crew, two crew member say to each other, “no way to tell, man.” “No way to tell from here.”
This is the epistemic overconfidence problem. It is true that in that moment, there was no way for the crew to know that the people being attacked by the Kiowa’s munitions were innocent civilians. In that moment, the claim that the families are terrorists is non-falsifiable because all of the information relevant to the decision has already been obtained. It has already been evaluated. And a false conclusion has already been drawn. The time to prevent the devastating mistake was not in that moment, but in the minutes and hours and maybe even in the years before.
What training had the Predator crew received to enable them, at each fork in the decision tree, to interpret the actions of the people on the ground accurately and effectively? At one point, the crew sees the families praying and acknowledge that jihadi terrorists likewise pray before an attack. They fail, however, to acknowledge that Muslims all over the world pray five times per day—even when traveling to market.
Fortunately, errors like this one have been quite rare in the US’s remotely piloted aircraft program, but that doesn’t make this error sting any less.
In the moment at which the crew was required to make a decision, they lacked the tools to interpret the information available to them. This is the problem of epistemic overconfidence and it is utterly relevant to the future AI in war.
The field of robotics has now delivered the power supplies, locomotive means, and control mechanisms to enable uninhabited machines to operate in the air, on land, on the sea, and under the sea (and, of course, in space—but that has been true since Sputnik).
Meanwhile, as robotics has been hard at work delivering hardware, the world of AI has developed in leaps and bounds in recent decades. I lack the space for a full accounting here. But one distinction in the history of AI is important to understand epistemic overconfidence.
2012 represented an important paradigm shift in the evolution of AI. In the preceding decades, most of the world’s leading AI theorists believed that advances in AI would come through deterministic software systems. Only a few, and perhaps most notably, Geoffrey Hinton, believed those advances would come through statistical methods. Hinton proved his detractors wrong in 2012 when his team, AlexNet, used statistical methods to develop an object classification, computer vision algorithm that, not only defeated all the other teams who were relying on cutting edge deterministic systems, but also showed real improvement in capability in a field in which improvement had begun to plateau.
Hinton’s win with machine learning in 2012 was the event that launched a thousand ships. AlexNet showed that, if given massive amounts of training data and computing power, statistical methods could out-perform deterministic systems at some computer science tasks.
This historical moment is relevant to so many features of our daily lives now—including the speech-to-text feature on your iPhone; the low latency rates in networked play on your gaming system; and my 2017 Subaru’s ability to sense the lane lines before nudging the steering wheel back toward the center. But Hinton’s achievement in 2012 is also relevant to war.
Imagine now a military drone in the not-too-distant future. Unlike many of the drones operating in Ukraine (and the US Predator and Reaper aircraft that predate them), this drone has a fully autonomous mode. It is capable of, say, departing from its base, flying to a pre-programed airspace, and then using statistical computer vision methods—based on the same approach that Hinton used to win the ImageNet challenge—to find a pre-programmed set of target classes. These classes might include adversary tanks, or military ships, or anti-aircraft artillery batteries.
Further suppose that this system is employed during an international armed conflict between two states; that the target classes that have been pre-programmed are strictly military objects and that, under the geopolitical circumstances, they are legitimate targets under the discrimination (sometimes called “distinction”) principle of both international humanitarian law and the just war tradition.
So far, this looks like a prudent employment of a novel weapons technology. The ethical challenge arises because, unlike in deterministic systems, the statistical systems that have exploded since 2012 are not encoded with rules for identifying relevant object classes; instead they are exposed to large datasets of the relevant object class and the models themselves determine which features—which patterns of pixel color and brightness—correspond with the object class.
We can imagine the system working well. Suppose the drone flies to its target air space, surveys the sea surface, and identifies an adversary military vessel, a member of the target object class. Further suppose that the drone conducts is kamikaze attack causing the maximum harm possible to the ships rudder, aiming to disable the crew’s ability to steer the ship. But in so doing, it kills two combatant crew members.
We can also imagine the system failing. Suppose the drone flies to its target air space, surveys the sea surface, and misidentifies a civilian fishing vessel as one of the military ships that was in its target class of objects. Likewise, this time the drone attacks the rudder, disabling the ship and killing two civilian crew members.
Success or failure in this scenario will not depend on knowledge of how Afghan families travel, or of how they carry their children, or of when they pray. It will depend instead on knowledge of the dataset on which the computer vision model was trained, and under what meteorological conditions, and whether the fidelity of the sensor produces images similar to those in the training dataset.
Mitigating these risks is a commander’s responsibility, to be sure. But commander’s will be positioned to manage these risks—in this case, risks both of mission failure and of inadvertently killing noncombatants—only if they have an adequate understanding of the technological capabilities of the system and the relationship between the training data and the real-world data.
This is not a technological challenge, but a cultural and organizational one. Militaries that intend to adopt AI-enabled targeting and autonomous military systems have an obligation to develop and deliver training and education to their future operators and commanders to ensure those operators and commanders can manage risk effectively; and that includes providing them with a baseline knowledge of how statistical AI systems work and how they can fail. Ultimately, this training and education should attempt to avoid the tragic situation in which the wrong people are killed and the human operators involved are left to look at one another and say, “no way to tell, man.” “No way to tell from here.”
*Disclaimer: The views expressed are those of the author and do not necessarily reflect those of the US Air Force, the Department of Defense, or the US Government.
Comments